Neural Networks Vs. Deep Learning
페이지 정보
작성자 Frank 작성일24-03-26 10:17 조회13회 댓글0건본문
Neural networks take much less time to train, however feature decrease accuracy when in comparison with deep studying; deep studying is extra complicated. Additionally, neural networks are known to interpret duties poorly regardless of quick completion. Deep learning is a posh neural network that may classify and interpret uncooked data with little human intervention but requires more computational resources. Neural networks are a less complicated subset of machine studying that can be skilled utilizing smaller datasets with fewer computational sources, but their capability to course of complex information is proscribed. Although used interchangeably, neural and deep studying networks are totally different. They've different strategies of training and degrees of accuracy. Nonetheless, deep learning models are more advanced and produce results with greater accuracy, as they'll learn independently with little human interference.
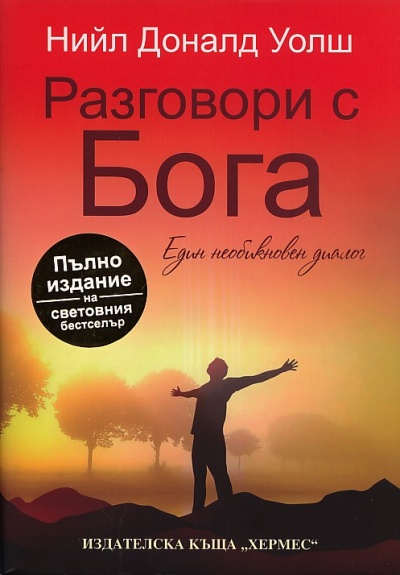
Companies and people must temper expectations in regards to the technology's capabilities, and take the time to completely perceive what AI can and cannot do, to allow them to truly select, pilot, and глаз бога телеграмм scale the technology successfully. As a shopper, you profit from AI in on a regular basis life. You hardly ever have to purchase AI instruments on your own. As an alternative, firms use AI to provide higher, extra worthwhile client experiences that find yourself serving you. Traveling problems with sales: It’s about determining the very best approach to get from one city to a different in a given space. Neural networks can deal with the problem of producing more revenue at decrease prices. Picture compression: It helps us to compress the data to store the encrypted form of the particular picture.
Finally, CNNs are utilized in pure language processing applications. Recurrent neural community (RNN): RNNs are deep neural networks that has the ability to store info from previous computations and passes it ahead so as to work upon this knowledge in a sequential method. They're a sort of neural community where the output of the earlier timestep is used as enter for the present timestep along with the new enter. This creates a relationship between the weather in the sequence, permitting the RNN to be taught dependencies between them. This makes RNNs nicely-suited for tasks reminiscent of speech recognition and machine translation, where understanding the context is crucial. Check out my blog on how RNN can be utilized for sequence to sequence modeling. RNNs will also be used for time collection prediction, corresponding to stock market movements or weather patterns. In recent years, RNNs have achieved important success on various difficult duties. Nonetheless, training RNNs can be difficult, because of the vanishing gradient problem. That is when the error signal becomes progressively weaker as it is propagated again by means of the community, making it troublesome for the RNN to learn from its errors.
Neural networks are becoming one of the vital related instruments for enterprise. They forecast demand and other factors, calculate patterns, automate many duties, together with interplay with customers, and plenty of different tasks that we will have a look at today. Taken together, AI solutions can optimize determination making, customer expertise, and efficiency across all industries. Scalability and future-proofing are also vital elements to consider when selecting an AI answer. With expertise continuously evolving, it is essential to choose a solution that can adapt and develop with your business’s needs. AI company ought to have a monitor file of providing scalable solutions and incorporating new technologies as they emerge. One other crucial consideration is customer support and ongoing upkeep. Implementing an AI resolution will be complicated, and technical issues may arise alongside the best way. TanH is much like sigmoid, but it’s a bit higher in terms of output accuracy. 1 and also includes destructive values. In the TanH perform, the bigger the input worth (more constructive), the closer the output is to 1. Equally, the smaller the input value, the closer the output is to -1. In the above-talked about formula, e stands for exponential constant.
댓글목록
등록된 댓글이 없습니다.